Have you decided that digitization is of strategic importance to your factory? Great!
But in what order should you approach this? What are the key steps? Above all, why do you want to do this in the first place?
If you are looking for answers, then you know how frustrating it is to read conflicting stories in the mainstream media. They pitch you one product after another, but the question of how remains basically unanswered.
Well, I’m going to explain that to you in this article. This 7-step formula gives you a constructive explanation of how to go about it.
Introduction
There are a few principles to remember before we can get started:
- Achieving Industry 4.0 does not happen at the push of a button. It is an evolution that will change the nature of how the metalworking industry and the rest of the world make products.
- A digital factory does not start by buying software or hardware. It starts with people and the organization, strategy and continuous improvement.
- By digitizing a bad process, it becomes a bad digital process. To be successful, you must eliminate waste continuous improve flow.
Now that you know this, let’s go over how to achieve a smart autonomous factory.
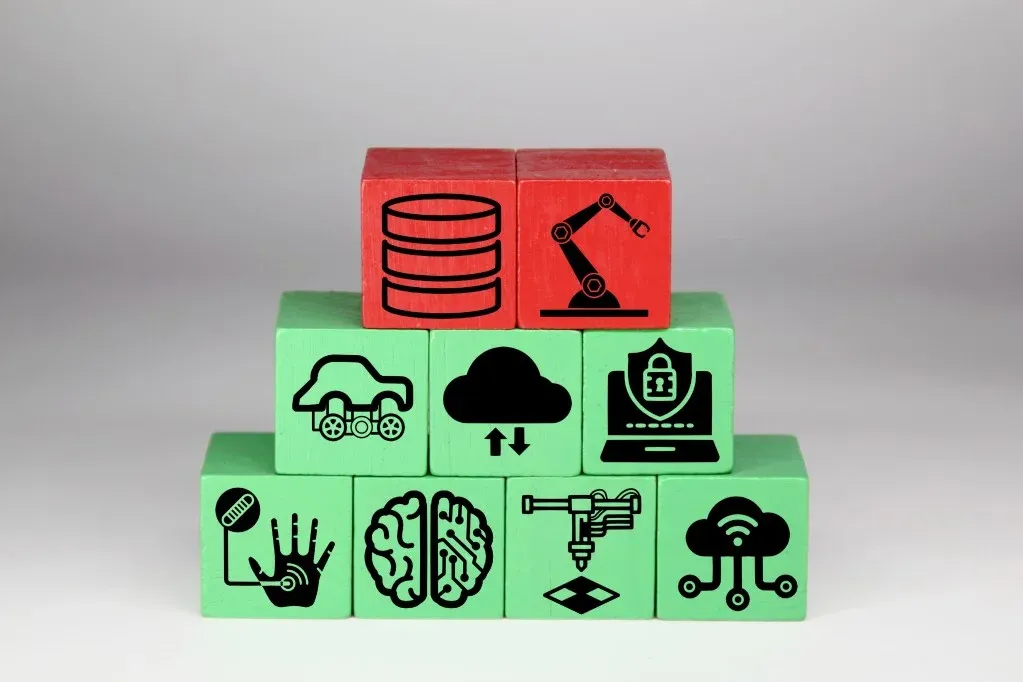
Lean Manufacturing, QRM and strategic measurement lay the groundwork.
Buzzwords such as the Industrial Internet of Things (IIoT), machine learning (ML) and artificial intelligence (AI) have been circulating among the professional and popular media for years.
Admittedly, I use them myself with some regularity. Apparently they are the future, right?
Well, automation will become autonomous, self-learning and self-correcting as people deal with exceptions. They will then process these problems and use them to further improve the process by then feeding information back to the system. Then the system learns from it which makes the process even better and exceptions even rarer.
The result: the plant can correct and manage itself and there are almost no exceptions.
“Okay, but what does that look like in metalworking, specifically for sheet metal?”
What can a progressive sheet metal worker do today to prepare for the autonomous future?
In this article, we navigate through the seven steps, with each step building on the others. The more robust those first layers are constructed, the more effective the later higher layers will be.
Let’s take an example: a sheet metal fabrication company with automated laser cutting, a comprehensive flexible manufacturing system (FMS), with automated warehouse towers and automation of unloading by robots.
At first glance, it seems that this business is well on its way to Industry 4.0 and fully autonomous manufacturing, right?
The answer to that question depends on how solid the foundation is laid by those first layers of Industry 4.0. The weaker those layers are built, the more likely a metal company’s efforts will falter as they progress.
So, what does the first step involve? Surprisingly, it does not focus on sensors, machines or even automation. Instead, it is about people and the organization.
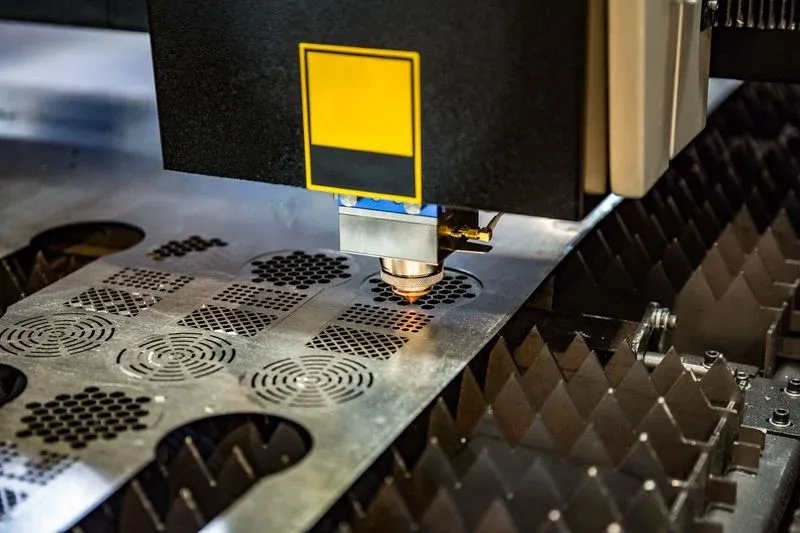
Step 1: Lean Manufacturing
You probably already know this saying, “garbage in, garbage out.”
What we mean by this is if inconsistent or illogical processes and practices are employed, then mainly leads to“garbage” data, at least from a high-level analytical perspective. It does not yet offer the insights you seek.
Of course, you can already uncover a problem and prove that the status quo is not good enough without needing much data to do so. The reason is that for the low-hanging fruit when it comes to problems, data from simple observations combined with a healthy dose of common sense can often suffice.
You can just walk around factory hall and collect data.
For example: You walk down the shop-floor and see work orders lying around without the materials there, people standing around the machine with a group talking about who did what wrong, and products that regularly go into the recycling container.
Another example: an operator of a combination laser cutting machine who constantly walks through the plant looking for plates and tools.
So both cases are clearly ineffective, and so you don’t need advanced analysis to clarify such a point.
Go Lean and Clean
Establishing lean manufacturing and quality rules is the first step.
You start by cleaning up the workplace, arranging it logically and making sure you have mutual rules for lean manufacturing.
Lean manufacturing involves applying 5S: Separate, Arrange, Clean, Standardizeand Support (or Sustain).
But it doesn’t stop there: you have to analyze the process from beginning to end.
Good analysis must also extend upstream, including plate ordering, material receipts and even supplier relationships.
Not inspecting incoming material and loading it into an automated storage tower without counting it is also a recipe for quality and inventory problems. Data analysis is not necessary to find this out.
Of course errors occur with operators, but here is the key:
Behind every error is a procedure that is wrongly followed, inadequate, documented or poorly communicated. Constantly perfecting those procedures lays the foundation for everything else.
Digitalizing an unstable process makes it more unstable.
Because: at least on paper you can see that things are still going wrong. You can see piles, there are trays, and you can look for it at each other’s workplaces, you can talk to people directly about that.
If you don’t handle digitization properly, you get contaminated, chaotic databases. And you won’t find anything in there.
If you want to achieve a digital factory as a company, you have to solve the right problems. And for most companies, the biggest problem is usually not the software, but the way they organize their daily business.
A metalworking company that does not obey the rules of lean manufacturing does not have a good basis for applying process improvement.
The data on which you then base process improvement are unclear or inconclusive. It can be distracting and even unhelpful if you made inference with this data.
So, it helps the most to solve those basic problems first, with common-sense and mutual agreements. The conclusion: If you digitize a bad process, it remains a bad digitized process.
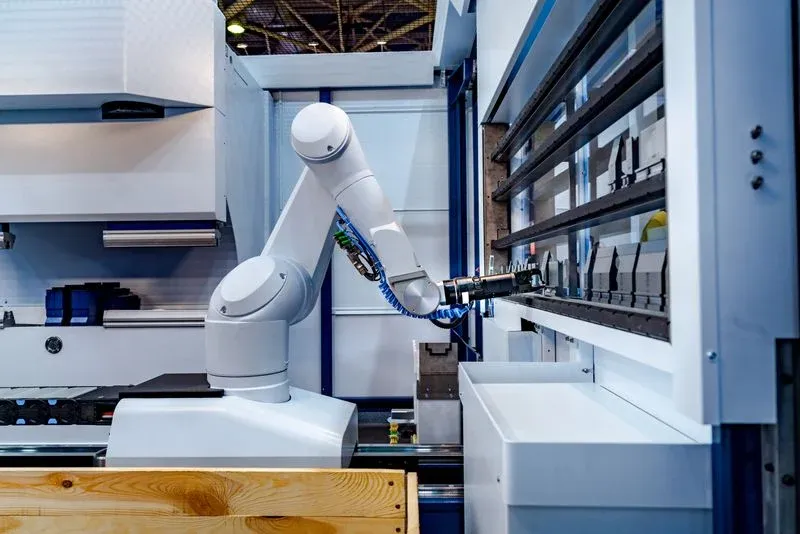
A foundation is a must
The path to Industry 4.0 is paved with seven steps or layers. The fundamentals are clear process agreements, a clear workplace and a logical layout of the factory hall.
Just imagine for a second, you’re building a skyscraper. What would it be like if you put 100 construction workers at work with no agreements on how work will be done, no one has to clean up, and everyone starts building in random places?
If a metal company determines that the digital factory is of strategic importance, you can’t just buy a software package and start building it with a team of countless consultants and IT people.
First, of course, most companies can’t afford that. But even if they could, it also creates chaos because projects often take too long and do not preclude the outcome of goals.
Therefore, they must first align people and organization.
The stronger the first steps, the more robust the later steps can be.
Conclusion: The first step is to set up the value stream so that there is little waste. Solving the low-hanging fruit is the foundation of a digital factory.
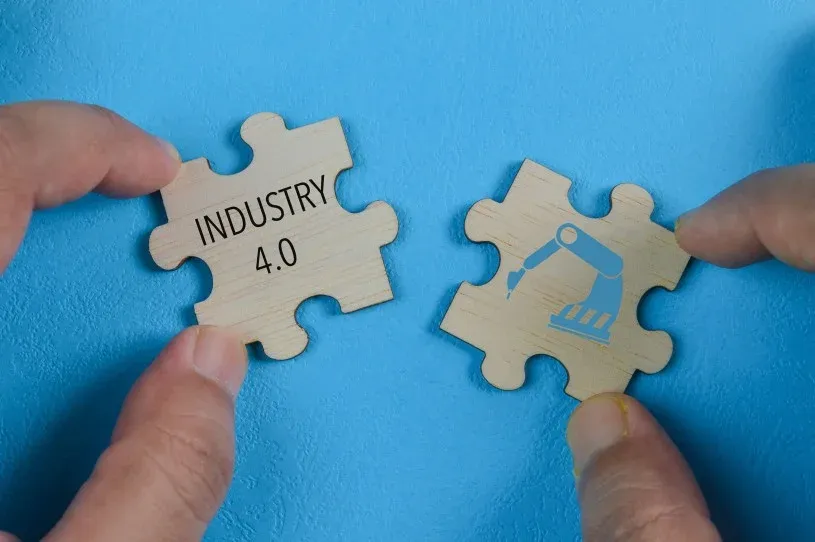
Step 2: Start thinking about time!
Note that as a sheet metal worker, you are not paid for machine uptime, but you get paid for the products you ship.
With the advent of the Internet, orders have become smaller, there is more customization and customers expect faster delivery. Time is therefore an increasingly important success factor.
So the faster you can deliver, the higher the selling price and the lower the cost.
QRM process improvement
That’s where Quick Response Manufacturing (QRM) comes in: the faster those products flow from raw inventory to shipping, the better.
Therefore, the main focus for today’s metalworker is: reducing lead times. The shorter the time in the factory, the less time spent searching, managing and the happier the customer is.
One of the biggest added benefits is that you also make organizations faster, more flexible and more effective. If you do it right, you can also achieve more output with the same number of employees.
For example: You can go for hefty batch sizes to make the overall uptime statistics (OEE) of the machine sky-high (the process then seems very efficient) thanks to shorter machine setup times, but: those huge batches will probably take a while to reach the shipping department.
In fact, there is a lot of waiting time between these steps, and local efficiency will be negated by the rest of the process. And you want to reduce those wait times to serve your customer with shorter lead times, a more reliable process.
Applying QRM is primarily an organizational change, but today it is also supported by digital processes. The most important thing here is to make the process stable: whether it needs to be fast or not, there are the same processes that were agreed upon beforehand.
Note: I repeat myself again: a process must be stable before you can optimize it on and from data-driven improvement. Without a stable (and enforced) process, most Industrie 4.0 initiatives will fail completely. QRM is also part of that and is not a one-time intervention.
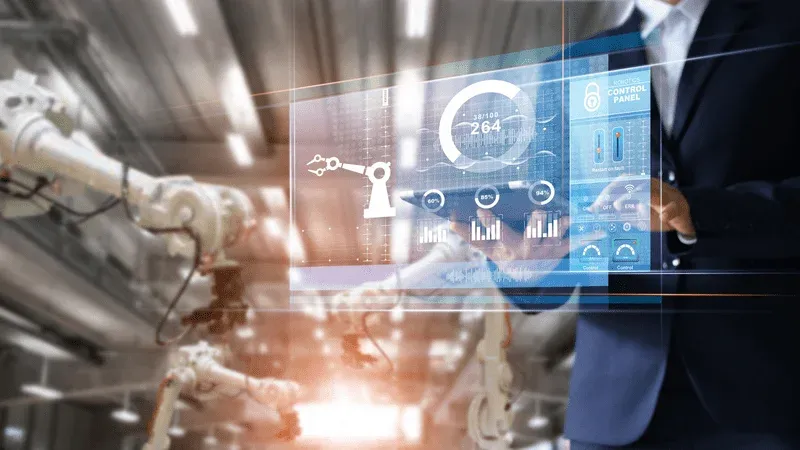
Step 3: Connectivity and Digitization
Only after you have done the 2 previous steps can you really start working with the software and machines.
The first step will be to digitize the work intake; I have written a separate article on that: “A digital factory starts with the work intake.
Practically speaking, this is in the process improvement associated with lean manufacturing: you eliminate waste, but of course, there are also many benefits to building accurate data.
When we mention the digital factory and autonomous systems, the main focus here will be on the factory floor itself.
Overall Equipment Effectiveness (OEE).
The first step in digitalization is to establish strategic metrics. For example:
- How active is the machine really?
- How much rework is there?
- What is going wrong with the machine?
Today, many modern sheet metal workers implement uptime tracking, where sensors report when a machine unexpectedly fails: the green-yellow-red mushroom on top of the machine, but to a spreadsheet.
Of course, being able to automatically notice when a machine that should be on is off is already great, but that’s just a first step.
What you actually want to measure is the Overall Equipment Effectiveness (OEE): loosely translated, the productivity of the machine, workplace and/or production cell.
Automated machines have multiple points for OEE measurements that can represent a larger process in greater detail.
For a laser setup, this can include loading and unloading of material, as well as various aspects of the laser cutting head’s behavior, as well as scheduled and unscheduled maintenance and even waiting logistics via AGVs.
What these measurements are, where they are taken, and how they take place depends on the machine design and the process involved. Anyway: OEE data – the kind that could form the basis for Industry 4.0 –
Such a process must be built from the ground up. For each process within a machine or system, you need a clear method for measuring OEE.
Another variable is data frequency, or how often a sensor (or human) picks up and delivers data in a useful format.
Each plant collects a series of data at different intervals. High-frequency data are collected in the moment, seconds or even milliseconds, and low-frequency data are often based on days. Intermediate frequency data are often collected on minutes.
These data frequencies must be matched with other process variables so that data analysis can compare apples to apples.
Data architecture
A system architect like me needs context to make data usable. A laser cutting machine can collect a lot of data points, but all that data means nothing unless you can relate it all to the actual cutting and order information.
You need to know which part was made on the machine, what information came from the Manufacturing Execution System (MES) and what the date and time stamp was.
Think of each data point collected by a sensor as a still image in time. You have all the intricacies of machine performance that the sensor collects, but you also have what the machine does – that is, the work it cuts.
With this data, you can start building patterns: how do certain jobs go through the machine so well and others don’t? Is there something wrong with the machine? Or could the problem lie elsewhere?
This, of course, is not limited to the laser machine, but also edgers, edgers and even welding machines.
Connectivity
Again, customers are buying products or parts, not machine hours. So one machine or system, even one the size of a modern laser cutting FMS, is only one piece of the puzzle.
You probably have multiple brands of machines (and software), and they all need to work together to exchange data. This is an important key and should not be underestimated.
What many companies don’t know is that most major machine builders have different ways of connecting systems together using common protocols such as OPCuA or MQTT.
Perhaps your supplier is not actively promoting this, but from experience I know that many manufacturers have a data connector that can help you further.
What is important here is that the data is stored uniformly in a logical location. Is the complete data set only on the machine controller, a separate PC or a dedicated database? What matters is that the timestamp is observed in the process.
There will be small time differences, and those time differences become extremely important as it relates to exactly when an error occurs and why
Either way, those analyzing the data need to know the timestamps so that everyone is on the same page. You start with the low-frequency data (days/hours), the basic actions, and gradually add more and more high-frequency data (seconds/miliseconds).
Shopfloor data
Importantly, operators also have access to a wealth of information. You want workers to record factory performance. Not every plant has an immediate need for timekeeping, given smaller batch sizes, but exceptions should always be recorded.
With a bending machine, for example, you want to know how long a product took to produce but also, more importantly, how the quality is in this production cell. This data should also be centrally stored and available in the larger data set you need in point 4.
Combining ERP and MES data
Once the machines are connected to the floor and there is complete shop floor data, it is important that the ERP and MES database is also open.
Just like through machines: through a standard interface such as API or SQL database , you want to link all this so that machines, software and process data can exchange.
If the part or order number is the same in all systems, you can then track this entire routing and also link geometry or edge information to OEE performance, ultimately making the data actionable information.
We address this in the next step. The more robust step 3 is, the more effective the upper levels become.
But beware: if the process is not stable and the measurements are incorrect or inadequate, everything else that comes next becomes difficult or even useless.
Next Level with Edge Devices
Some processes require immediate action. This means immediate intervention on the line. It is not enough here to wait for analysis that may take minutes, hours or even days. This is where edge and cloud computing come into play.
What is edge computing?
Edge computing leverages the power of algorithms as you would normally run it from a cloud server, but on a local computer or operating system.
Examples of such a use case include a video feed of a camera inspecting cut parts as they exit the laser machine, where robots then automatically pick up the cut parts and place them on a conveyor belt.
You can then have another robot automatically sort specific products, label them or laser engrave a QR code. This QR code then contains all the product’s digital information, which comes from the ERP or MES. This includes what material was used for tracability purposes.
And, of course, you can perform quality control fully automatically if discrepancies are found.
As you can imagine, this is almost impossible to do when all this data first goes to the cloud, then has to be sent back to the machine, and all in real time.
Then you need to switch to a computer with the vision-inspection machine learning model connected directly to the camera.
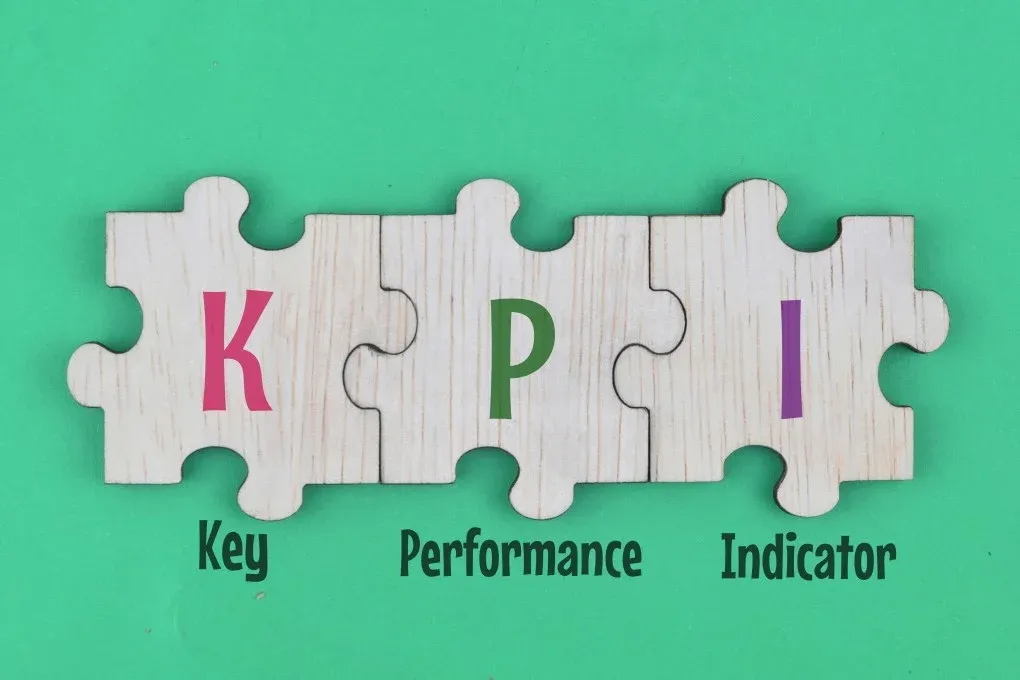
Step 4: Data to Information
Data is not information. Information is the answer to a question asked.
You can’t have information without data.
Things only get done when the data we collect can inform and inspire those in a position to make a difference.
Data by itself cannot lead to change or improvement, but improvement can occur after the data has been analyzed, contextualized and translated into actionable information.
Making productivity measurable
The following is inspired by Eli Goldratt.
Productivity is the art of bringing a company closer to its goal. Any action that brings a company closer to its goal is productive. Any action that does not bring a company closer to its goal is not productive. The goal is to generate more output to generate more money
At this point, you have collected a lot of data, but no connections that lead to information yet. Only now can you start applying data analysis principles and start applying OEE (productivity) analysis.
You see the potential output of a machine, workplace, team or the entire plant. You know what it could do in theory, and now it’s a matter of finding the gap between the potential and the current result.
For example, the machine or workplace produced good parts, bad parts and some parts that are unknown (good or bad).
Bad parts mean it is wrong, or took much longer, or caused logistical problems.
The goal is to eliminate the unknown, increase the good and minimize the bad. Now the analysis information from good and bad parts can relate to other process information.
Some of this initially comes from a manual search (“query”) that you have to come up with yourself: you write your own formula for this or have to formulate a specific query.
You can then get the answer directly from the machine, machine/ERP software or a data warehouse.
But don’t forget, last but not least, the machine operator/operator!
The operator’s gold
As mentioned earlier, machine operators are well informed: they observe the process and may have made process notes about cutting behavior or part jams or other improvements for nesting strategies that the programmer should know about.
However, the latter information should not be taken as the only truth. You should add this information to related data recorded over time – cutting conditions, production information and machine logs, etc.
Eventually you arrive at the “holy grail,” a complete database with a digital record of every production process and every part produced.
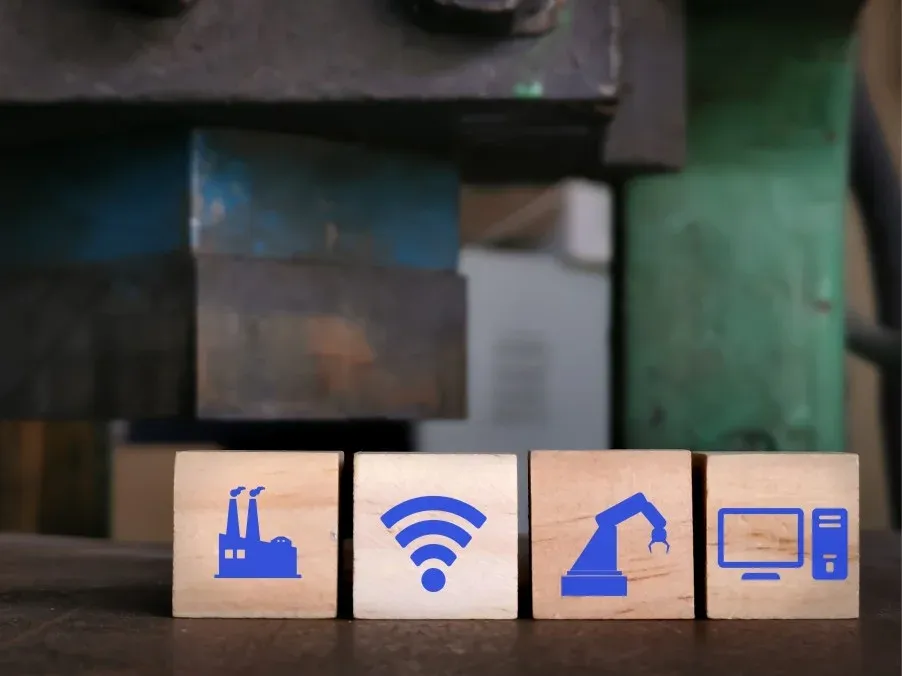
Step 5: Company-wide Knowledge
Once we can link information to events and use it to reach a conclusion, that is knowledge that becomes fundamental to continuous improvement.
This knowledge can then be documented in a database or other system where people can retrieve it and use the solution to solve similar problems.
The team needs to see
If only two people in an entire company have access to information and can use it to solve problems, the likelihood of anyone doing anything with it is very low. Ideally, you want to share this more broadly.
Everyone, or at least as many trained employees as possible, should have access to the data — whether in an ERP, MES or another system such as a BI tool — and be trusted enough to analyze the data to solve problems or improve processes.
As information gathering becomes more automated over time, the knowledge gained in step 5 becomes more automated. Eventually, automated control begins to take on more and more autonomous properties.
Go big with data
The more knowledge a system has, the better the data sets and inputs, the greater the potential for further automation with machine learning.
And the ideal is that the digital knowledge base of the machine and the process grows, absorbing so much data and information that the analytics achieved with machine learning can determine solutions that humans would not have otherwise considered.
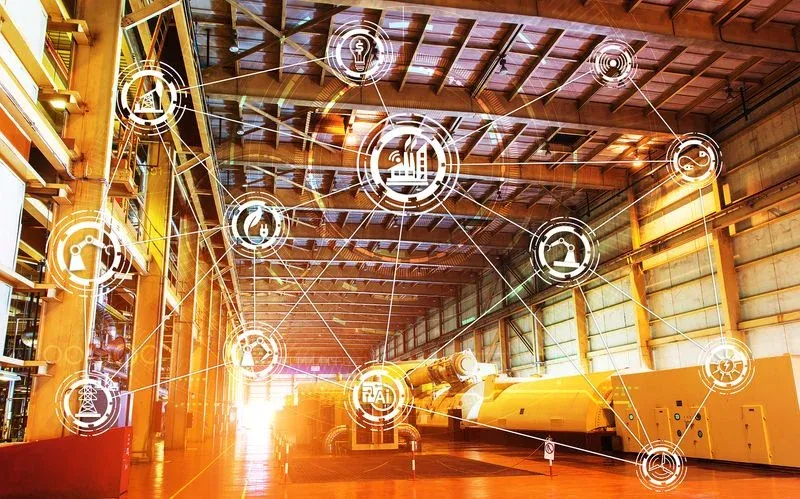
Step 6: Predictive Analysis.
If you do everything right so far, both in the office and in the workplace, you get machine learning done: you can start predicting the future and seeing from where you’re going.
The goal is that this way you can prevent problems before they arise.
You will see if machines are still functioning correctly, but also especially when they are in danger of malfunctioning. Perhaps you can prevent tolerance problems before they happen.
You will begin to see patterns in material consumption and when shortages may be imminent. You can then also see when the probability of this is greatest.
A good prognosis will tell you.
Prediction must be learned
These analyses will initially be manual, with IT professionals analyzing current data and interpolating with software to predict future performance.
However, as machine learning evolves with more and more real-time data capture and analysis, the systems themselves can make these forecasts and suggest corrective actions.
As a manager, you can capitalize on that before it’s too late. You could even go so far as to automatically shut down processes when certain criteria are met.
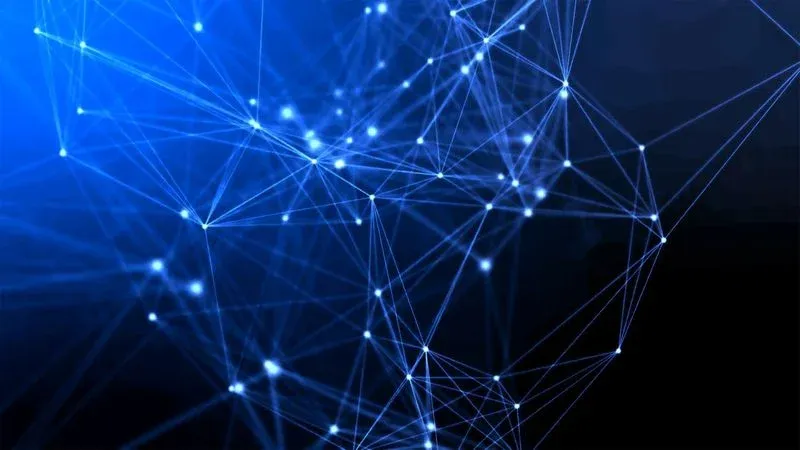
Step 7: Full autonomy
We have arrived at the “holy grail”!
If you tackle everything according to the principles of industry 4.0, this is where true maturity is achieved in full implementation.
Unlike the popular talk of a smart factory that machines are connected to each other, directly across the entire production line from lasering to bending, this is a bit more nuanced.
In a true smart factory, machines are connected to the network and linked to all information systems.
Machines not to each other, but 1 central source of truth
All data collected in the factory must be sent to the brain of the organization. In most cases, this will be the MES system, which stores all production data.
Better yet, a dedicated data warehouse where information from other systems such as ERP can also be added. This central database can then conveniently organize the data for analysis, so you can go in all directions here.
As a result, the factory of the future will be able to respond much more flexibly to changing demand. All connected systems can adapt accordingly. Production needs can be adjusted on the fly , self-directed logistics (AGVs) independently support the shop floor, and even tools and materials are automatically placed in the right place.
An example: AGVs can deliver a stack of laser-cut workpieces to an automated press brake, which, after scanning them, opens a connection to the MES and networks the smart algorithms, the factory’s “brain.
This system reveals product and customer information from the MES, as well as data from upstream machines that was shared when those machines processed the part.
It also reveals information from the video system that detects the actual component on the AGV. Knowing all this, the brain instructs the press brake to take the best possible action.
Autonomous adjustment of process
One thing is certain: something always goes wrong. In a smart factory, however, this need not be a problem because you can adjust this automatically.
Perhaps the cutting process variables were slightly off, or perhaps the part was nested on a ground plate but accidentally cut in the X direction instead of the Y direction.
Because of the heat input during laser cutting, the cut piece may not be completely flat, the piece may still be stuck in the plate with a large burr, or it may still have inner contours that did not come out properly.
All these things are pre-detected by automatic recognitions. The machine will then be able to determine if this part can still be machined further, or if re-work is required.
If you can go further, such a bender customizes the bending process by adjusting everything from the robot’s bending sequence and gripper selection to the paths the robots take to orient and shape the workpiece.
In an autonomous world, machines will configure themselves. And the software will anticipate the adjusted demand around that.
From intake to delivery
An autonomous factory is not just about what happens on the shop floor. In a competitive market, many companies will be able to make the most of their profits in the pre-production process: engineering, sales and production preparation.
Again, the same benefits and approach apply: we connect all systems, including the rest of the chain (suppliers and customers) and then perform analysis on this data.
The more data and better searches, the smarter the system gets until it can finally adapt itself. If handled properly, digitization helps exchange data between automation systems without human intervention. Even management by exception is becoming increasingly rare.